Table of Contents
The United States reacted to the COVID-19 pandemic in an unexpected way—along mainly partisan lines we either relied upon or expressly rejected "the science." The two main camps:
You can't trust anything the government tells you. Period. Definitely including all the fake science
In an emergency like this, making stuff up and ignoring the science can only lead to needless death, suffering and costs. You have to be an idiot to reject the expert scientific views.
Even well-publicized deaths among vocal critics of the reliability of science-informed public health advice failed to much check the rejection of the scientific resources.
I still don't believe in the science, I have no faith in it.
was a widespread sentiment as was its antithesis.
As the crisis deepened the patterns of transmission and infection produced data that could be analyzed to inform policy decisions. Some long-held consensus beliefs had to be reversed on closer study. The leading example was that airborne transmission of virus particles were of concern only in the immediate vicinity of an infected person. The rationale was that respiratory vectors were encapsulated in liquid droplets that would only travel a short distance before falling to ground. This seems to have gone into the six-foot social distancing rules. The virus particles didn't agree they needed droplets—it eventuated that aerosols suit very well. Coughs and sneezes were dangers that could be distanced from because they were easy to detect. Ordinary exhaling of the moist breath we normally produce turned out to have a much greater effective radius. Because of latency between becoming infected and manifesting symptoms the danger cannot be spotted.
On the whole, consensus opinion grumbled when they had to reconsider what they thought of as "settled" and change direction. But it happens because the nature of science is that it is provisional pending what can be a very long period of observation, analysis sorting factors that appear to be associated from those that appear to be unrelated. Or, indeed, finding consistent associations between factors that seemed odd fellow travelers.
Even then, with the cycle of see, measure, test and think may yield only a "we are not sure exactly why we see this, but … ." The well-worn adage "correlation is not causation" bears on this difficulty. Statistical causation is feasible but it requires painstaking work to control for possible head fakes arising from confounding variables. Methods such as directed acyclic graphs can help tease out relationships that have some direct bearing. A popular account is The Book of Why by Judea Pearl.
Provisionality induces mental discomfort which undermines the sincerity that supports assessment of credibility. The surveillance approach discussed below shows evidence of a coping measure that bears the indicia of a well rehearsed tactic often described as "arm waving."
This chart (shown at the top of the page) from The New York Times free link caught my eye. The $x$-axis is time and the $y$-axis is the number of standard deviations above a baseline low, defined as the 10th percentile. The purpose is to estimate trends in COVID-19 prevalence based on wastewater sampling.
Following links from this CDC page I'm bothered by
- Presence of viral RNA is established from three positive droplets measured by RT-qPCR or one when multiple assays or multiple PCR replicates are run. This seems skimpy.
- Normalization is allowed be based on estimates of the served population. From my past personal domain knowledge utilities did not collect information at the household level on number of residents.
- Observations showing no virus are recorded as half the detection level for no stated reason.
- A minimum of only 3 data points is required for trend estimation.
- Trends are identified by the slope of the linear regression, which ignores time series autocorrelation issues in regressing observations of log transformed SARS-CoV-2 normalized concentration against "date"
I don't have to speculate what Richard Feynman make of this methodology because it so clearly falls into a category he illustrates
In the South Seas there is a Cargo Cult of people. During the war they saw airplanes land with lots of good materials, and they want the same thing to happen now. So they’ve arranged to make things like runways, to put fires along the sides of the runways, to make a wooden hut for a man to sit in, with two wooden pieces on his head like headphones and bars of bamboo sticking out like antennas—he’s the controller—and they wait for the airplanes to land. They’re doing everything right. The form is perfect. It looks exactly the way it looked before. But it doesn’t work. No airplanes land. So I call these things Cargo Cult Science, because they follow all the apparent precepts and forms of scientific investigation, but they’re missing something essential, because the planes don’t land.
Cal Tech 1974 Commencement Speech
What I think of as Sewergate (actually sewer grate) is an analysis that purports to provide what the PowerPoint™️ crowd likes to call actionable stuff—reasons you can point to for taking or refraining taking some policy action to affect the course of events. In this case, paying attention to sewage provides insight into trends of prevalence of the virus in urban populations before cases begin to enter the health care system.
Forewarned is forearmed
is hard to argue against, especially when the warning is based on well observed, well tested and highly replicable analysis. But, really
We can never know about the days to come, but we think about them anyway. Carly Simon
For afficiandos, here is the gory takedown of sewergate.
On the overall approach
There are several potential issues that can arise when attempting to estimate the trend over time by using linear regression to calculate a slope:
- Non-linear trends - If the underlying trend is accelerating, decelerating, or follows a curved trajectory, fitting a straight line can give misleading results about the true rate and nature of change over time.
- Non-stationarity - A time series needs to be stationary (constant mean/variance over time) in order for a simple linear regression trendline to be valid. For many real-world data phenomena this is not the case. Using a non-stationary time series can throw off the linear regression assumptions.
- Outliers & noise - Extreme outlier observations or excessive random noise can distort the least-squares linear fit used to calculate slope. This makes the trend estimate unreliable.
- Extrapolation error - Projecting a linear regression line too far outside the available historical time window is subject to increasing inaccuracy. There can be lower confidence that future data will follow historical trends.
- Explanatory factors - There may be cyclical, seasonal, intervention, policy, environmental or other explanatory factors changing over time in addition to overall trend direction. A simple univariate regression cannot account for these, missing potential causal influences on the evolution of the time series when quantifying trend slope.
Prudent usage of linear regression trend slopes requires first checking that underlying assumptions hold reasonably true and having awareness of the potential limitations in accurately capturing secular change over time. Augmenting with models that can account for other explanatory variables is recommended where feasible.
Specific criticisms
Yes, using a log-transformed and normalized concentration as the outcome variable in a linear regression trend analysis introduces some additional complexities:
- Re-transformation: Taking the slope of a log-transformed regression and multiplying by the mean response is needed to get an accurate trend estimate in the original units. Failure to apply this re-transformation properly can result in a biased trend.
- Magnitude obfuscation: Logging compresses larger values and expands smaller values in a nonlinear way. This visually obscures the true magnitude of changes over time, making the trend slope harder to interpret. Translating back into the original concentration units helps comunicate the practical significance.
- Relative change: By normalizing to an assumed source population value, the resulting concentration is now depicting proportional or percentage change rather than absolute change. The trend slope then reflects the rate of percentage change rather than raw concentration change, further complicating contextualization.
- Model assumptions: Taking the log converts a multiplicative error process into an additive one. So the linear regression assumptions apply to modeling the logged data. However, assumptions may still be violated in the original untransformed space.
Overall, extra care must be taken when inferring trends from logged or standardized data to ensure accurate re-transformation, meaningful interpretation, and avoidance of guideline extrapolations.
Feynman's philosophy on "cargo cult science" and self-delusion, attempting to estimate trends from only 3 observations over time via linear regression bolsters the case for skepticism of the science, rather than faith in it. A few reasons why:
- With so few data points, it becomes much easier to "fool oneself" into seeing patterns that may not truly be there or extrapolating well beyond reason. The uncertainty bounds are extremely wide.
- Three observations make it basically impossible to detect most violations of assumptions like non-linearity, non-stationarity, autocorrelation of errors, etc. that could invalidate the simple straight line regression model.
- Sampling variability could heavily influence the slope estimate and direction if an outlier happens to be one of the 3 observations. No crosstabling with other variables is possible to check explanatory factors.
- Reporting a precise numerical slope value and making confident projections implies an certainty that is likely not warranted based on n=3 data points scattered in time. More humility in interpretation would be prudent.

The estocada
When a bullfighter finally plunges the sword into the back of the bull searching out his vital organs to deliver a death stroke, the culmination of the matador's skill and the nearing of the end of the bull's cruel suffering is the estocada. This is supposedly a sublime, almost spiritual moment that elevates what would otherwise be a display of sadism into a mystical melange of contemplation of skill, courage, grace and the mortality facing every living being. I sat in the arena in Seville one very hot day and watched a succession of rookies as graceless as they were inept at the end. I walked out in disgust across the board.
Feynman would harshly critique putting too much stock in the validity or generalizability of any patterns inferred from only three temporal observations. While the math can provide a precise linear slope statistic, the philosophical spirit of science calls for much more humility and explicit doubt in such limited data situations. Fostering skepticism is wise, rather than suggesting firm or even useful conclusions.
Impossible to maintain a straight face
Look again at the highlighted line at the top of the plot first shown, purporting to represent 25 deviations from a 10th percentile baseline. Does it pass the "laugh test" to be taken seriously from a scientific or statistical perspective? No, and here are just the highlights.
- Reaching 25 standard deviations is an astronomical anomaly that essentially never happens in natural phenomena or data. It implies something has gone very wrong with the data collection, analytics, or the validity of using a normal distribution.
- The probability of seeing a 25 sigma event randomly if the data were actually normally distributed would be essentially zero - far less than 1 in a billion. It is numerically suspect.
- The fact it is benchmarked against the 10th percentile further suggests it is an extreme outlier observation compared to 90% of the data. This makes it almost certainly spurious.
- No physical, chemical, biological mechanisms could plausibly explain or account for an event or observation departing a whole 25 standard deviations from baseline. The laugh test probes basic intuition about how mechanistic systems work.
Overall, any reasonable scientist would be incredulous and demand extraordinary evidence before accepting a claim about observing a 25 𝛔 deviation relative to baseline natural variation. Even Jack Welsh was able to scrape by with only six. It contradicts both statistical rigor and scientific common sense. At best it reflects a faulty analytical method - at worst outright fabrication. Subjecting it to extreme skepticism is prudent rather than taking it at face value. It does not pass the laugh test. Take it to open mic night, mate.
Je regrette énormément
It is not to be expected for a lead investigator to appear bef0re a committee of elected or appointed officials who have sponsored an expensive study of an untried approach to managing an important policy challenge and saying
In conclusion, we have developed some useful refinement of lab technique, learned how to deal with challenges relating to uniform collection practices and uniform reporting of results from decentralized study partners.
We did not, of course, ever hope to be able to reach definitive conclusions based on this pilot study. In retrospect, I must confess that we over-reached by elaborating what can only be charitably called fudge factors to overcome limitations imposed by the number of available data points and the low levels of virus we were attempting to detect. This much is to be regretted and for this the responsibility must rest with me.
In light of these deeply disappointing results, I am withdrawing our Phase II funding request. While this will leave many bright and motivated graduate students without support for the coming year and/or leave them with too little for dissertations, I cannot in good conscience continue this line of research.
In The Sun Also Rises, Earnest Hemingway's hardbitten newsman Jake Barnes dismisses an expression of sadness expressed from Lady Brett Ashley that they will never become lovers and how wonderful that would have been. Unstated but understood is that Barnes is impotent due to his war injury. In the final line of the story he says
Isn't it pretty to think so?
Mascot of the Day
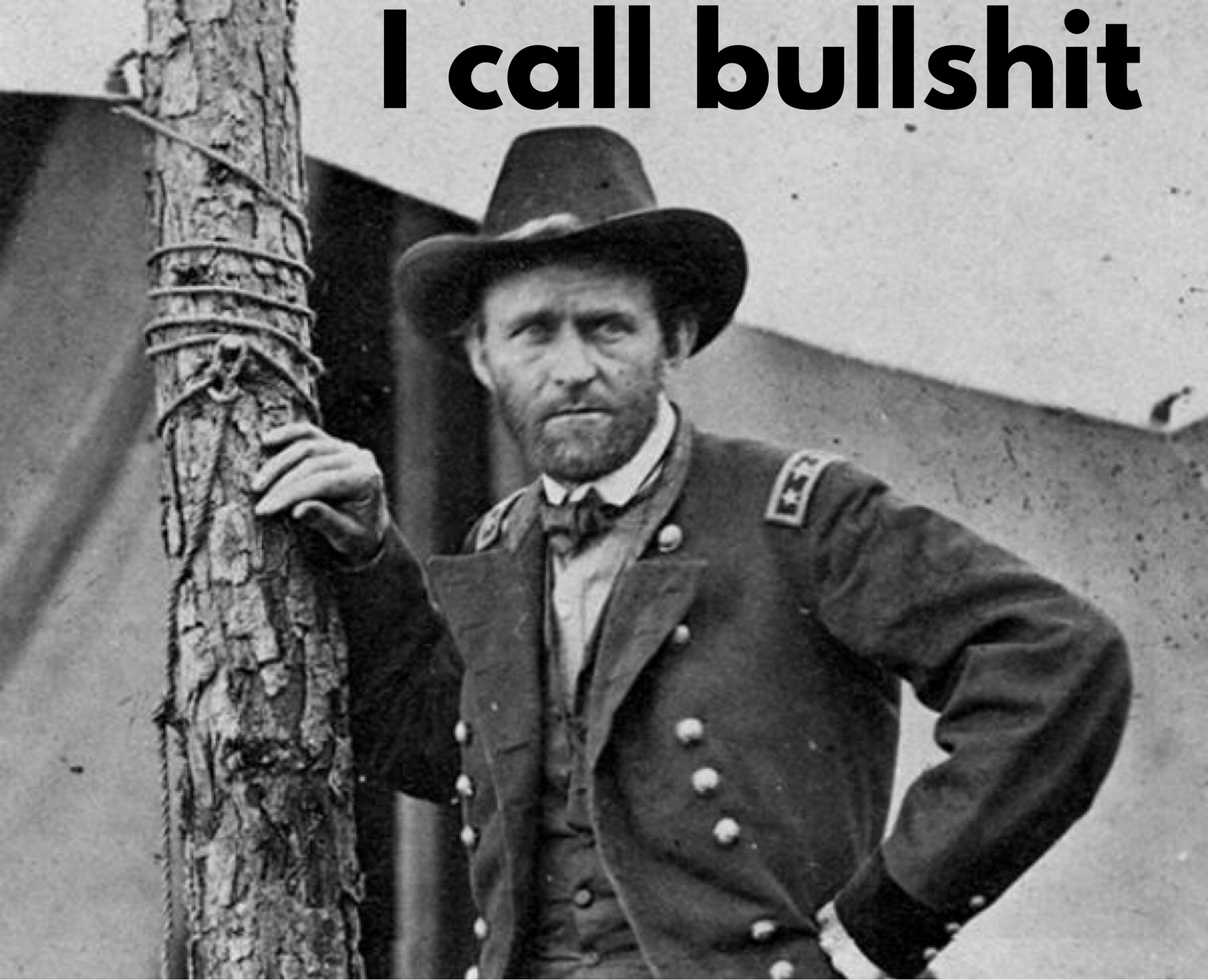